Financial Statement Fraud Detection in the Digital Age
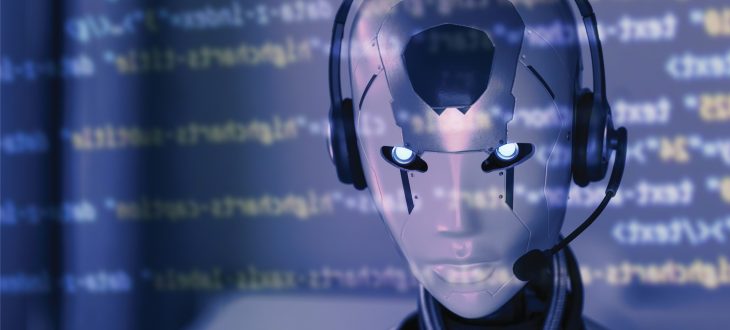
The proliferation of technology throughout modern business has created novel opportunities for financial statement fraud. But technology tools can also be leveraged to help detect and prevent fraud. Contemporary artificial intelligence (AI) approaches have the potential to be more efficient and accurate in detecting fraud, especially novel frauds. But […]
The proliferation of technology throughout modern business has created novel opportunities for financial statement fraud. But technology tools can also be leveraged to help detect and prevent fraud. Contemporary artificial intelligence (AI) approaches have the potential to be more efficient and accurate in detecting fraud, especially novel frauds. But although AI models can analyze volumes of data too vast for humans to handle, they still rely upon human intuition, experience, and analysis to train them and look out for bias and error. Successful implementation requires careful planning, investment, and expertise.
***
The digitalization of the global economy has created unprecedented opportunities for various kinds of frauds, some of which may involve, or create a corollary need for, financial statement deceptions. The proliferation of technology—and its accelerated adoption during the COVID-19 pandemic—has changed how companies conduct business and perform services. This has increased the need for innovative controls and other processes to protect against such risks. Fortuitously, technology—which may be one of the greatest enablers of frauds—also provides tools to prevent and detect their occurrence.
The Prevalence of Fraud
Financial statement fraud involves the intentional creation of false or misleading information in financial statements. Such frauds are commonly perpetrated by owners or managers to overstate financial profitability or viability, or to conceal actual theft. This is the costliest category of occupational fraud affecting organizations, according to the Association of Certified Fraud Examiners’ (ACFE) 2024 Report to the Nations (https://legacy.acfe.com/report-to-the-nations/2024/). According to the SEC, improper revenue recognition, reserves manipulation, and inventory misstatement are among most prevalent of such schemes, with CFOs (54%) and CEOs (31%) most frequently the perpetrators (SEC, “New Report Reveals Common Themes in SEC Enforcement of Financial Statement Fraud,” January 12, 2021).
Corporate failures and scandals across the globe continue to call into question the role and responsibility of auditors for timely detection of fraud, as well as for instructing clients on matters of prevention. The following are notable examples of corporate failures where fraud was a factor:
- Wirecard AG (2020), a German payment processing company that inflated its revenue and profits to deceive investors and lenders, filed for insolvency in June 2020 after admitting that €1.9 billion ($2.1 billion) had disappeared from its balance sheet. Its former CEO and other executives have been charged with fraud, market manipulation, and other crimes.
- Luckin Coffee Inc. (2019), a Chinese coffeehouse chain, was accused of fabricating more than $300 million in sales transactions that sent its stock price plummeting. This led to the resignations of its CEO and COO, delisting of its shares, investigation by the U.S. SEC, and multiple lawsuits.
- Steinhoff International Holdings NV (2017), a global furniture and household goods company, announced that its CEO had resigned in the wake of the discovery of “accounting irregularities,” including billions of dollars of fictitious or irregular transactions over several years.
- Toshiba (2015), the Japanese electronics company that had acquired the troubled Westinghouse nuclear power construction business, was found to have inflated its profits by $1.2 billion over several years by improperly booking anticipated profits and delaying recognition of losses. This led to the resignation of the company’s CEO and other top executives, and fines by regulators in Japan and the United States.
As a result of those corporate failures, financial losses were not only incurred by investors, lenders, employees, and other stakeholders, but there were also wider costs to society, including a lessening of confidence and trust in auditors and regulators and in the perceived integrity of financial markets, resulting in higher transaction costs and reduced market efficiency.
Fraud has historically represented a great business risk for the independent and internal auditing professions because both are perceived (rightly or wrongly) to be the most directly responsible for establishing internal controls that would prevent or detect fraud. Major CPA firms have previously reported spending as much as 20% of gross fee income on litigation, settlements, and insurance (B.J. Epstein, “When a Triangle Becomes a Singularity: Assessing and Responding to ‘Dark Triad’ Fraud Risk in the Audit Environment,” 2019 Williamsburg Advanced Fraud Academy, Williamsburg, Va., May 9-10, 2019). Auditing standards (most recently, the AICPA’s SAS 99 and PCAOB’s AS 2401) and the federal securities laws have addressed auditors’ responsibilities with regard to fraud detection, and yet external and internal auditors detect only a limited number of fraud incidents (at rates of 4% and 15%, respectively—ACFE, 2024).
In March 2023, the PCAOB issued a proposed new standard, AS 1000, General Responsibilities of the Auditor in Conducting an Audit, citing “advancements in technology affecting the availability of electronic audit tools and use of audit software.” The IAASB has been examining disruptive technologies for their effect on audit and assurance services, to be able to respond appropriately to enhanced risks created by technology.
The IAASB is considering whether the financial statement audit should include procedures that are more forensic in nature, which raises the potential need for auditors to receive training in forensic auditing [IAASB, “Proposed International Standard on Auditing 240 (Revised): The Auditor’s Responsibilities Relating to Fraud in an Audit of Financial Statements and Proposed Conforming and Consequential Amendments to Other ISAs,” https://tinyurl.com/yv5kxyye, February 2024]. As many have long-since observed, forensic examination techniques are not qualitatively distinct from audit procedures, but rather occupy a point further along an existing continuum of testing strategies designed to support financial statement assertions.
Recent exponential increases in computing power and statistical modeling facilitate countering fraud in real time. Artificial intelligence (AI) and machine learning have created or improved upon various fraud detection algorithms, constituting a step up from traditional rules-based approaches that were more time and effort consuming and that resulted in more false positives.
Recent exponential increases in computing power and statistical modeling facilitate countering fraud in real time.
Artificial Intelligence
AI is a field of computer science that focuses on the development of machines and systems to perform tasks that normally require human intelligence, such as learning, problem solving, and decision making. AI can be a powerful tool for detecting financial statement fraud by analyzing patterns and anomalies in financial data, identifying potential fraud risks, and predicting new and emerging types of financial fraud. But AI models must be trained on copious quantities of relevant, high-quality data, and continuously monitored to ensure accuracy and effectiveness.
AI encompasses a wide range of techniques, including machine learning, natural language processing, robotics, computer vision, and expert systems. These techniques allow machines to analyze large amounts of data, learn from experience, and make decisions based on changing patterns and obliquely altering rules.
Machine Learning
Machine learning (ML) is a subset of AI that involves developing algorithms to recognize patterns in data and making predictions or decisions based on perceptions about those evolving patterns. Proper machine learning techniques can distinguish fraudulent activities from legitimate behaviors. Although they are often presumed to be impervious to common human biases because they operate on data and algorithms, machine learning tools can still reflect the human biases and the prejudices of their creators, making this supposition inaccurate.
There are several types of machine learning (ML) algorithms, each with their own strengths and weaknesses. The most common types of ML programs are for supervised learning, which involves training a model on a labeled dataset (e.g., used to detect financial statement fraud), and for unsupervised learning (which includes clustering and anomaly detection). Other types of ML algorithms include natural language processing and deep learning, which is often used for image and speech recognition, and other applications that require complex feature extraction. The variant of machine learning to be employed depends on the specific problem to be addressed and the available data.
Natural Language Processing
One key advantage of AI models is their ability to analyze unstructured data, such as text and images. Financial statements often contain textual information, such as footnotes and the management discussion and analysis (MD&A), which may provide valuable insights into potential fraudulent activities. Structured data—provided in spreadsheets and ledgers—can be analyzed using data analytics and automated systems; yet more than 80% of data today is in unstructured formats such as contracts, emails, PDFs, and other documents [J. Rizkallah, “The Big (Unstructured) Data Problem,” Jun. 5, 2017, https://tinyurl.com/44cxa4jj]. A key challenge is to develop digital tools that can read this “big data” and identify relevant information.
Natural language processing (NLP) is the subfield of AI that deals with the interaction between computers and human languages, focusing on unstructured data. NLP attempts to address the inherent challenge that, while human communications are often ambiguous and imprecise, computers require definite and exact messages to enable understanding (I.E. Fisher, M.R. Garnsey, and M.E. Hughes, “Natural Language Processing in Accounting, Auditing and Finance: A Synthesis of the Literature with a Roadmap for Future Research,” Intell. Syst. Account. Finance Manag., vol. 23, pp. 157-214, https://api.semanticscholar.org/CorpusID:205966938, 2016). NLP focuses on enabling machines to understand, interpret, and generate human language by teaching them how to process and analyze large volumes of text data. It is increasingly valuable for coping with the explosive growth in unstructured data, including email, text messaging, audio, and video. It can help parse what is being conveyed in troves of such data, information that could give the lie to the assumedly reliable more structured data, thus signaling fraudulent activities. The difference between technology and humans performing this is one of scale—an NLP application could read thousands, even millions, of documents in a fraction of the time it would take a human to complete the same task (KPMG, “Dynamic Audit Technology Content Series: Natural Language Processing Point of View,” 2021, https://tinyurl.com/u42ed5eu).
NLP algorithms can be used to peruse financial statements, including the notes and the MD&A sections, to identify any unusual language, wording, or patterns that may indicate fraudulent activity or misrepresentations. NLP algorithms, when combined with robotic process automation (RPA), can analyze 100% of revenue or purchase transactions, facilitating judgments about areas of risk given potential outliers and exceptions. Although this is a critical step in the audit process, auditors must still consider the reliability and relevance of supporting documents (e.g., invoices) to determine the appropriateness of the audit evidence (KPMG, 2021). For example, NLP was used by one company to flag a spreadsheet entry showing that a particular order was being procured using a standard product code for industrial goods, although notes accompanying the order revealed that nonqualified items, such as televisions and laptops, had been included in the transaction.
NLP algorithms can be used to analyze financial data in real time, which can be useful in detecting fraudulent activity as it happens. For example, NLP algorithms can be used to monitor social media platforms and other online sources for any mentions of a company’s financial performance or indicia of potential irregularities that might signal a need for prompt investigation. NLP was also used to review customer contact center audio files to determine if agents pressured customers to buy products in excess of current needs (encouraging “channel stuffing,” a common fraudulent technique), analyzing agents’ tone of voice and customers’ stress levels. NLP, including “voice stress analysis,” can also help identify connections between people who otherwise have no known links, by analyzing similarities in their comments and other speech characteristics.
NLP can also be used to analyze other types of financial data, such as news articles, social media posts, and other online content. By examining these sources, NLP algorithms can identify information that may pertain to the company’s financial health, such as news about potential mergers or acquisitions, changes in leadership, or other events that could impact the company’s financial performance. Indeed, the wider the range of source materials used to train the NLP or other AI algorithms, the more accurate they will become—the true essence of machine learning protocols.
NLP algorithms can be used to peruse financial statements, including the notes and the MD&A sections, to identify any unusual language, wording, or patterns that may indicate fraudulent activity or misrepresentations.
The development of Large Language Models (LLM) has revolutionized the field of NLP, and these tools can also be used to help detect financial statement fraud. LLMs are computer programs that use deep learning algorithms to process and generate human-like language. These models are trained on vast amounts of text data and can perform a wide range of language-related tasks such as text classification, question-answering, language translation, and text generation. They are widely used in applications such as chatbots (e.g., ChatGPT), virtual assistants, and language translation systems.
Natural language processing (NLP) has been used in various ways to detect financial statement fraud, including the following:
- Sentiment analysis is a technique used to determine the emotional tone of an item of text—for example, negative sentiment in certain language used in financial statements may indicate that a company is trying to conceal negative information such as financial losses or declining sales within overall positive language about financial performance and prospects, which could be a red flag of a cover-up.
- Keyword analysis is used to identify specific words or phrases commonly found in fraudulent financial statements (e.g., restructuring costs or one-time charges that might be used to obscure the true nature of expenses).
- Named entity recognition is used to analyze the relationships between different entities mentioned in financial statements, such as companies, products, or key personnel, in order to identify potential instances of financial statement fraud based on these unacknowledged relationships.
- Topic modeling is used to detect word and phrase patterns, such as those dealing with revenue growth, expenses, or investments, which could indicate fraudulent activities, such as manipulating revenues or expenses.
- Latent semantic analysis is used to analyze relationships between words and concepts in a body of text, to identify patterns or anomalies in the financial statements—for example, frequent mentions of revenue growth not translated into higher profit or cash flow, which could suggest areas for further investigation.
The need for the involvement of trained personnel even when tools such as NLP are employed should not be underappreciated. The use of such approaches is enhanced dramatically through human involvement in the process, a key component of all forensic analytics. Experienced, knowledgeable people can both pursue investigations based on the analytics and provide feedback on its utility and effectiveness, enhancing investigative capabilities over time.
Data Mining
Data mining (DM) involves using statistical and machine learning techniques to extract meaningful information from large sets of data. DM is often used in conjunction with other AI techniques, such as ML, NLP, and computer vision, which enable AI systems to learn from data, reason about complex problems, and make intelligent decisions.
Exhibit 1 presents a two-layer conceptual framework, a structured approach to the application of data mining to financial statement fraud detection. The first layer comprises the six data mining application classes of classification, clustering, prediction, regression, visualization, and anomaly detection. The second layer sets forth different application algorithms to extract the relevant relationships in the data and present the results in a visual format that will aid decision making. The two layers are different, relatively independent, self-contained, and mutually supportive.
Exhibit 1
Conceptual Framework for Application of Data Mining to Financial Statement Fraud Detection
Source: Based on the financial crime framework of the U.S. FBI, updated from a secondary source (A. Sharma and P.K. Panigrahi, “A Review of Financial Accounting Fraud Detection based on Data Mining Techniques,” International Journal of Computer Applications, vol. 39-1, 2012)
Data mining techniques and their applications in financial statement fraud detection include the following:
- Classification—the process of building a model or algorithm that can predict whether a financial statement contains fraudulent information or not, based on various financial ratios, trends, or other indicators, using specific classifiers such as random forest, decision trees, neural networks, and Bayesian beliefs networks.
- Clustering—an unsupervised machine learning technique that groups similar data points together based on their features and characteristics, which can be useful for detecting financial statement fraud. Some of the cluster models are K-nearest neighbors (KNN) and K-means clustering DBSCAN (density-based spatial clustering of applications with noise).
- Prediction—analyzing trends and patterns in financial data to identify financial statements that deviate significantly from industry norms, thus warranting further investigation. For example, models can be developed to predict the likelihood of future transactions being fraudulent, identify areas of risk within an organization, or identify customers who may be engaged in fraudulent activities (see “Predictive Analytics” below).
- Regression—this technique is used to identify financial statement fraud by identifying outliers, predicting fraudulent behavior, analyzing trends in financial data, and selecting the most important variables for predicting fraud, using, for example, logistic regression (LR) and linear discriminant analysis (LDA).
- Visualization—another set of techniques designed to detect financial statement fraud by identifying outliers, analyzing network relationships, identifying trends in financial data, and selecting the most important variables for predicting fraud. Graphical representation involves presenting data in a visual format (e.g., charts, graphs, diagrams) to help detect patterns, anomalies, and trends in the data. A network diagram of related-party transactions may reveal a complex web of transactions between related parties, suggestive of fraudulent activity.
- Anomaly detection—a powerful tool for detecting financial statement fraud by identifying unusual transactions, outliers, patterns in financial data, and fraudulent behaviors. ML algorithms, such as neural networks, decision trees, and natural language processing, are examples of such techniques.
Clustering methods include transaction clustering, financial ratio clustering, entity (e.g., customers, suppliers) clustering, or text clustering (e.g., MD&A, footnotes, disclosures), all of which can be helpful in identifying fraudulent activities. Density-based spatial clustering of applications with noise (DBSCAN) works best when there are large datasets and the number of clusters is known in advance.
An Example of Clustering of Revenues for Overstatements or Understatements
Exhibit 2 presents an example of how the DBSCAN clustering method can be used to identify overstated or understated revenues by identifying patterns or anomalies in revenue data that may indicate fraudulent activity. The first step is to gather the necessary data (e.g., financial statements or sales records) and their relevant features (e.g., growth rate, volume, profit margin) for analysis; then the data can be subjected to a clustering algorithm, based on such information as whether the transaction date (and invoice) and shipping date match (the occurrence assertion), whether the quantities and product codes on invoice and shipping documents match (accuracy), or whether the total sale on invoice and cash receipt match (occurrence and accuracy). This algorithm will group similar data points together and identify instances that do not fit within the established patterns. These outliers (anomalies) may represent over- or understated revenues that are outside the norm of typical revenue patterns. The identified anomalies or outliers would then need to be investigated further to determine whether they represent actual cases of over- or understated revenue, which may involve reviewing financial records, conducting interviews, or performing other forensic accounting techniques.
Exhibit 2
DBSCAN Method Applied to Identify Overstated or Understated Revenues
Notes:
- The middle cluster is the revenue data meeting the criteria; the right cluster represents potential overstated revenues, and the left cluster represents understated revenues.
- Noises are outliers in the statistical terminology. While collecting data, humans tend to make mistakes and data points tend to be inaccurate, so the collected data has some error bound to it. Noises are not considered in this analysis.
- Core Points are the average amounts in that cluster.
- Border Points are the potential fraud points in the cluster.
Robotic Process Automation (RPA)
Robotic Process Automation (RPA) can be a powerful tool for detecting financial statement fraud by automating data analysis, continuous monitoring, reducing manual errors, and enhancing internal controls. RPA “bots” can perform tasks such as data entry, data extraction, and data processing with greater accuracy and efficiency than humans, improving the accuracy of fraud detection. In addition, RPA can enhance internal controls by automating financial data analysis and flagging potential issues, helping organizations identify and address fraud risks more quickly and effectively.
Although data mining and RPA are separate tools, they can be used together to improve business processes and decision making. For example, data mining techniques can be used to identify patterns and gain insights into large data sets that can then be used to automate routine tasks using RPA bots. Similarly, RPA can be used to collect and analyze data from multiple sources, which can then be used for data mining and analysis to develop insights and observe trends.
Predictive Analytics
Predictive analytics, a subset of data analytics, entails the use of statistical and machine learning algorithms to examine historical data and make predictions about future events or behaviors. Data analytics encompasses a wider range of techniques and processes, including data mining, data cleaning, data transformation, exploratory data analyses, descriptive analytics, and predictive analytics.
Predictive analytics can be used to identify financial statement fraud by identifying anomalies in financial data, applying trend and ratio analysis to discover unusual patterns, or using text mining to identify any unusual patterns or other red flags.
There are numerous business intelligence software tools available for data analytics. The following are some of the most popular ones:
- Tableau, a data visualization tool that allows users to create interactive dashboards and reports.
- Microsoft Power BI, which provides interactive visualizations and business intelligence capabilities.
- Alteryx, a data analytics and automation platform that provides predictive analytics capabilities and includes ML algorithms.
- RapidMiner, an open-source data science platform that provides predictive analytics capabilities and includes machine learning algorithms.
- Python, a powerful programming language that has become increasingly popular for data analytics due to its simplicity, versatility, and large library of data analysis tools.
Application programming interfaces (API) can be used to automate the process of detecting financial statement fraud by providing access to financial data and using ML algorithms to identify and prevent suspicious activity.
AI vs. Traditional Methodologies to Detect Financial Statement Fraud
AI approaches to financial statement fraud detection use ML algorithms to learn from past examples of fraudulent and nonfraudulent financial data. These algorithms can automatically detect patterns and anomalies in the data, without relying on predefined rules, and thus can be more effective at detecting new and previously unknown fraud schemes, adapting to changes in the data and fraud landscape over time. In addition, AI can analyze large volumes of data more quickly and accurately than human experts can do manually. Detecting fraud earlier and more efficiently reduces an entity’s financial losses, and the ability to analyze unstructured data furthers the potential savings.
Traditional rules-based approaches to financial statement fraud detection rely on a set of pre-defined rubrics that are programmed to detect specific patterns or anomalies in financial data. These rules are typically based on expert knowledge and experience, and they require human intervention to update or modify the rules as new fraud schemes emerge. Although traditional rules-based approaches have been effective in detecting known fraud patterns, modern AI-based approaches promise more accurate and efficient fraud detection, particularly in the face of evolving fraud schemes and increasing amounts of financial data.
Exhibit 3 summarizes the key differences between traditional rule-based approaches and modern AI approaches to financial statement fraud detection.
Exhibit 3
AI vs. Traditional Methodologies of Financial Statement Fraud Detection
Examples of Risks Associated with Emerging Technologies
Although using emerging technologies to detect financial statement fraud can offer several benefits, several attendant risks should be evaluated, including the following:
- Risk of overreliance: the use of technology may potentially create biases, such as a false sense of security, leading to a general risk of overreliance on technology and on the output of the audit procedure performed, without considering the human element in fraud detection. Overreliance on technology can be the cause of, or result from, less attention or emphasis on professional judgment, experience, or professional skepticism (L. Harris, “The Hidden Dangers of Machine Learning-Based Scams,” ACFE, Jan. 6, 2023).
- False positives: the use of AI and data analytics to detect financial statement fraud may result in false positives, wherein legitimate financial activities are flagged as fraudulent. This can lead to unnecessary investigations, increased costs, and reputational damage.
- Limited training data: AI and data analytics rely on historical data to identify patterns and anomalies. If there is limited training data available, however, the accuracy of the models may be compromised.
- Data quality: the effectiveness of AI and data analytics is highly dependent upon the quality of data used. If data quality is poor, the models may not be able to identify fraudulent activities accurately.
- Cybersecurity risks: the use of technology can expose financial data to cyber-security risks, such as hacking and data breaches.
- Ethical concerns: the use of AI and data analytics in fraud detection raises ethical concerns around privacy, bias, and the potential for unintended consequences.
In short, although the use of emerging technologies can enhance fraud detection capabilities, it is important to carefully assess the risks involved and develop appropriate risk management strategies.
Proceed Carefully
Modern AI-based approaches can offer more accurate and efficient fraud detection than traditional rules-based techniques, particularly in the face of evolving fraud schemes and increasing amounts and complexity of financial data. A key advantage of AI models is their ability to analyze unstructured data, such as text and images, to identify key terms and phrases that may indicate fraudulent activities. AI models also have limitations, however, such as the need for high-quality and comprehensive data to train the models and the potential for bias or errors in the models. Traditional approaches to detect financial statement fraud often rely on human intuition, experience, and analysis of historical data. Many believe that the financial statement audit should evolve to include procedures that are more forensic in nature.
Although emerging technologies offer significant potential for detecting financial statement fraud, organizations must be prepared to address the challenges involved in implementing these technologies effectively and ethically. This requires careful planning, investment in resources and expertise, and a commitment to data quality, privacy, and security.
Karina Kasztelnik, PhD, is an assistant professor of accounting at the college of business at Tennessee State University, Nashville, Tenn.
Eva K. Jermakowicz, PhD, CPA, is a professor of accounting at Tennessee State University, Nashville, Tenn.
Click here to view full article